We love helping HR teams achieve their goal to be more data-driven. So we try our best to learn from the most passionate HR data analytics advocates, like Giuseppe Di Fazio, Director of People Analytics & Workforce Planning at Silicon Valley Bank, specializing in data architecture, workforce analytics, and people operations. With 10 years of experience in HR across various industries, his insights had us glued to the screen during our live, interactive webinar entitled “ShockwaveTalks: Cleaner Data for Reliable People Analytics”.
In this article we lay out Giuseppe’s step-by-step guide with slides and video snippets from his ShockwaveTalk!
Why does clean data matter?
Whenever huge amounts of data are processed and modelled from various sources, mistakes are likely to occur. This leads to a greater chance of using corrupted, duplicate, or inaccurate data in workforce analytics. HR teams that deal with data must be aware of this and must understand that Data Cleansing is essential to reliable people analytics, as “incorrect data can lead to false beliefs, assumptions and insights, inform poor decision making and damage trust in the overall analytical process,” according to Giuseppe.
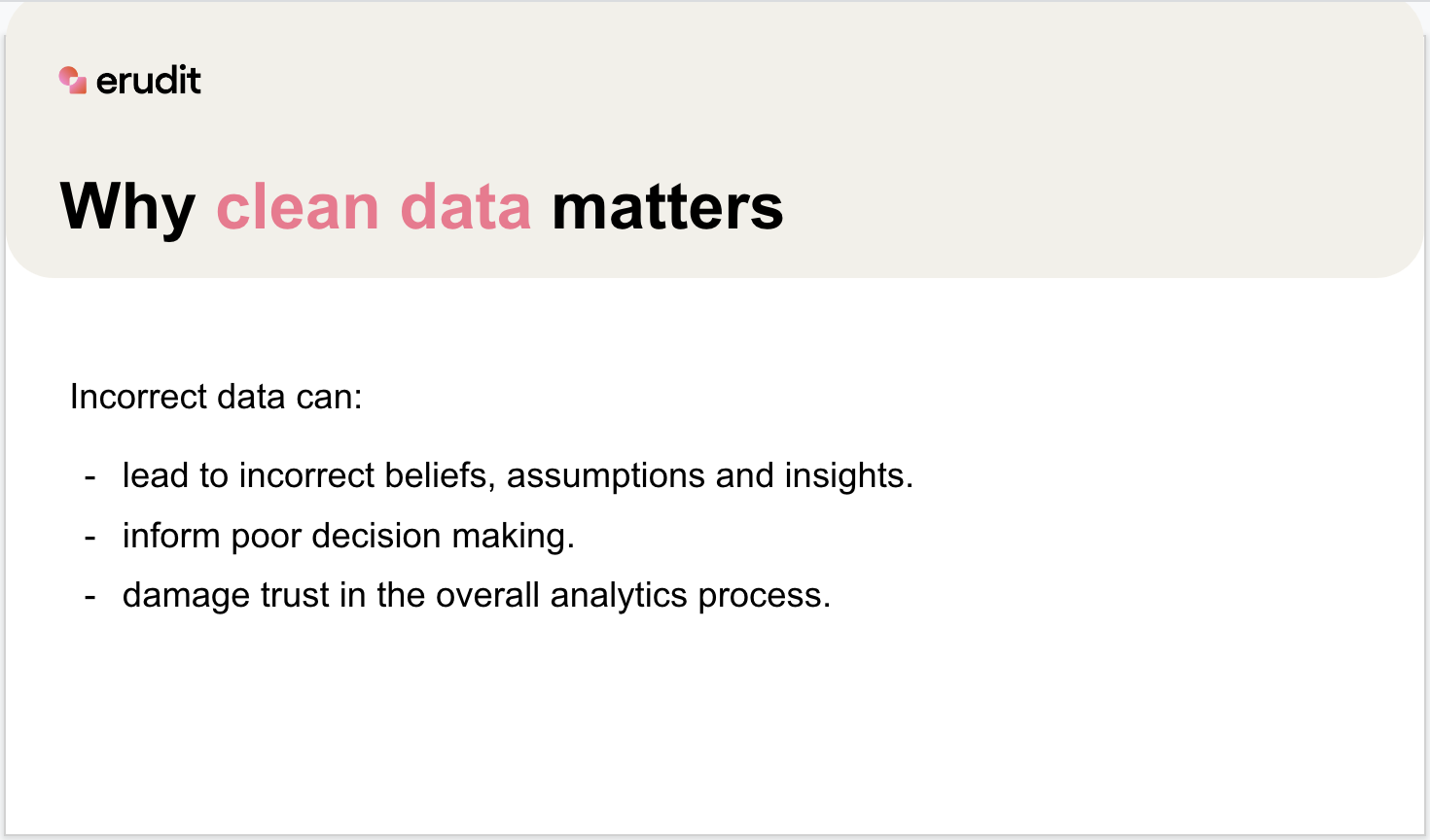
What is data cleansing and what’s the 3-step process?
Data cleansing is the process of removing the following types of data within a data set:
- incorrect
- inaccurate
- incomplete
- inconsistent
- duplicate
- incorrectly formatted
It can become dirty through user error, poor communication, and coordination across departments or inadequate data strategy and processes.
So, to avoid flawed data, the analytics superstar presented a three-step process for data cleansing:
1. Data assessment. We have to look at the data and ask: Where does it come from? How was it used? What technologies were used to collect it? Which processes and who were involved? How was the data entered and managed?
2. Data remediation. This involves fixing the errors found in the previous step.
3. Data monitoring and auditing. Once the data is 99% clean, we need to put processes in place to make sure that data stays clean.
"Incorrect data can lead to false beliefs, assumptions and insights, inform poor decision making and damage trust in the overall analytical process."
The dictionary for data assessment, a must-have tool
Before we get our hands dirty with data cleansing (See what I did there?), let’s make sure we have the proper tools to dig in. The data should be standardized, meaning that the same definitions and formats apply to the data gathered, even when taken from different sources. Every data-driven organization must build their dictionary or glossary which documents all agreed-on definitions, terminology, formats, and other conventions for the data. All the different stakeholders should take time to prepare this as it is the tool that will guide you through the data cleaning process. This dictionary includes:
Applicable to all elements:
- Simple and consistent naming conventions (Will we call it “Turnover Annualized Rate” or “Annualized Turnover Rate”?)
- A consistent formatting approach (date format, number of decimals, etc.)
Applicable to each element:
- Detailed definitions
- An explanation of how they are calculated
- A valid output range (valid data labels for categorical data, valid data ranges for numerical data, etc.)
Listen to Giuseppe's tips on how to build your own data dictionary or glossary!
Data remediation: Where to look when cleaning the data
Giuseppe identifies four major categories and recommends acting on them in chronological order:
1. Remove duplicates. Chances of having duplicate data are high when using many sources. Therefore, removing duplicates should be the first step of the cleaning process, which will shrink the size of the data before moving to the next steps. However, duplicates might not be evident until after the data has been formatted, so Guiseppe considers this step as the first but also the fifth in the cleansing process.
Be careful! Some data might look like duplicates if we use just a handful of fields, so we might need to consider adding more fields for the analysis to see if any of the assumed duplicates actually have a reason to be there.
2. Fix structural errors. In this step, you will examine typos, formatting, homogeneity in the terms used to name the same data, and conventional terminology - do we use "non applicable" or "N/A?"? A perfect example of when the use of the aforementioned dictionary is essential.
3. Fix outliers. This is where we discuss acceptable ranges for each of the matrix values. We also talk about when there is a conflict in the logic of some of the fields. Sometimes, despite making sense individually, fields have ranges that conflict with each other.
Guiseppe mentions the example of someone who is marked as part-time in the part-time/full-time field, but then in the scheduled hours per week they have 40 hours, which in the US means full-time. Outliers of this kind can be fixed, but there are others that do not need fixing. For example, when an employee resides in one country and gets paid in the currency of a different one. This is usually a mistake, unless we are talking about an expat.
4. Missing data. Giuseppe suggests contacting the person responsible for the specific data in order to find out why this data hasn't been collected. This will enable you both to figure out together how to deal with this error.
Sometimes, other connected data can be used to fix the missing data. For instance, if an employee got a raise and you don't have the effective date, but you do have data about their promotion. In this case, it’s very likely that both events are connected and you can use the date of the promotion for the former missing field.
People Analytics expert, Giuseppe Di Fazio, goes through each step with examples.
Auditing your clean data
There are four concepts you should apply when making sure data is clean and stays clean:
Validity.
Is the data confirming our rules and constraints? Are we measuring what we need to measure? How did we measure it? More than having clean data, it is about asking ourselves if we really have what we need to take action on it. Were we clear when collecting data? Were we consistent?
Accuracy.
Is the data close to the true values?
Completeness.
Are all the required data known? This concept might vary. For example, in the US the estate of residence is needed in order to get an employee’s full address, but this same field might not be applicable in some other countries because it is not needed for the full address. It is important, then, that the definition of completeness is the same across different departments within the company in your data dictionary.
Consistency.
Is data consistent within the same data set? Across different data sets? Is the data collected annually or year-to-year? Sometimes the way things are measured changes, scales change all the time. Therefore, we have to check if we are consistent in the way the data is gathered, maintained and presented.
Conclusion
There you go! Now you can build your people analytics on reliable and clean data thanks to the concepts and step-by-step process as explained by Silicon Valley Bank’s people analytics rockstar, Giuseppe Di Fazio.
Still craving more tips? Watch the full talk and Q&A or follow Erudit on LinkedIn for bitesized insights from data-driven HR professionals who rock!